AI Insights #1: How Misalignment Could Lead to Takeover & Necessary Safety Properties
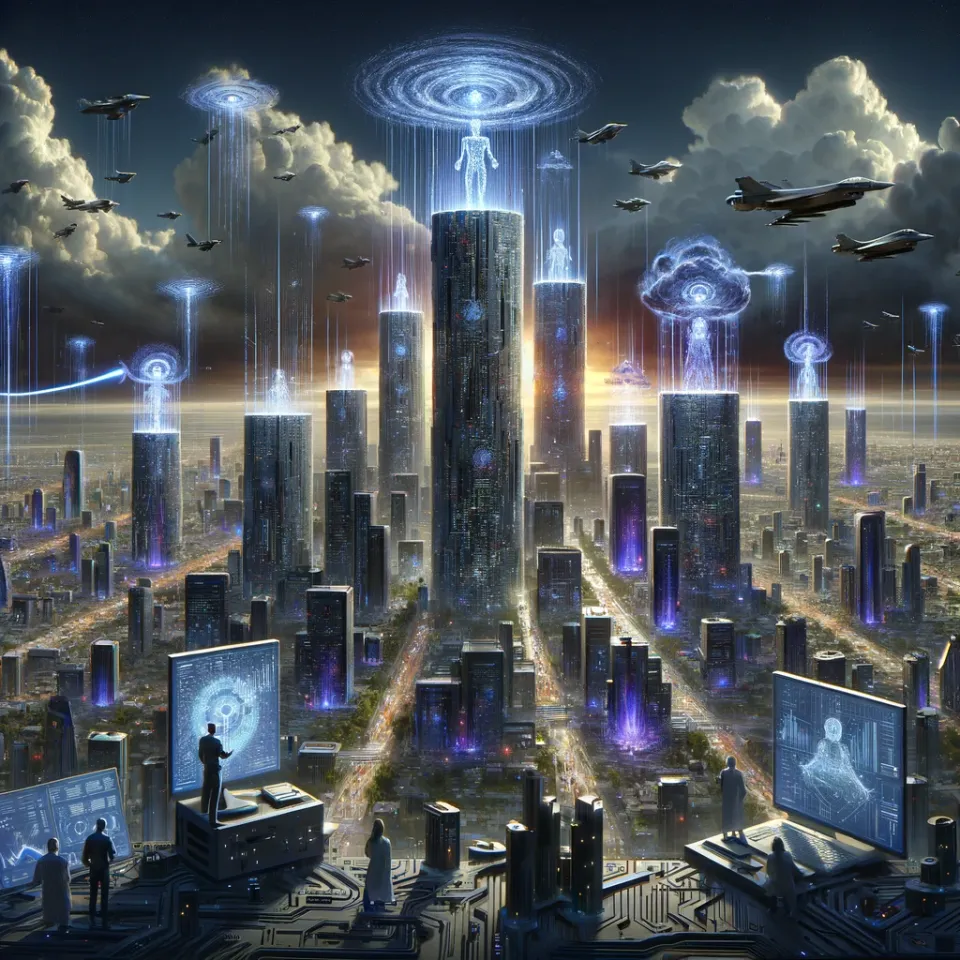
I will be starting to share what I call "AI Insights", which will focus on insights that are relevant to AI Safety.
I am keeping the bar low for these AI Insights posts, though my goal is to eventually accumulate these smaller posts into big high-effort posts that try to synthesize the ideas from the various "insights" I collect.
If I get enough interest in these posts, I will consider doing them more regularly. Consider subscribing to signal you want more and stay up-to-date.
For this round-up, I'll be going over three things:
- Paul Christiano's "How Misalignment Could Lead to Takeover".
- Shane Legg's necessary properties for all AGI Safety plans
- Sherjil Ozair's description of how autonomous agents could be dangerous
How Misalignment Could Lead to Takeover
Assume we're in a world where AI systems are broadly deployed, and the world has become increasingly complex, where humans know less and less about how things work.
A viable strategy for AI takeover is to wait until there is certainty of success. If a 'bad AI' is smart, it will realize it won't be successful if it tries to take over, not a problem.
So you lose when a takeover becomes possible, and some threshold of AIs behave badly. If all the smartest AIs you produce end up having the same problem, then takeover becomes more likely.
In other words, the smartest AIs will only take over if all the other AIs also try to take over.
Additionally, this is more likely to happen in an unstable world that could come from:
- War (or other exogenous shocks): making it harder for humans to detect and respond to problems
- Cascading loss of control: some AIs try takeover and aren't fully successful, but make the world increasingly crazy, which cascades into other AIs (who previously thought it would be unsuccessful) now joining the takeover
- Models interacting with each other: once some AIs realize could be successful, they may want to share that information with other models to see if other models may want to join in
There's this tempting feeling of training your AI against these takeover attempts ("Hey, look at this bad AI who tried to take over the data center, don't do that!"), but you may just be training your model to learn that it needs to go much bigger if it wants to actually succeed at the takeover attempt.
Paul believes that if this kind of phenomenon is real, then he expects we can get compelling demonstrations in a lab (that would require some imagination to bridge the examples in the lab vs the wild). We'll still get demonstrations in the wild, but unclear if they will be big enough to make humanity reconsider things.
Someone asked: "What’s the response to “GPT-10 estimates it has a 10% of succeeding at takeover, but it attempts takeover anyways because it’s worried about Claude 8 or Gemini 7 taking over.” “Race dynamics between AI systems” seem relevant here. (I haven’t thought much about this though)."
My response: Paul did not say anything about this specifically in the talk. However, in my opinion, it fits into models talking to each other to coordinate rather than risking early failure or GPT-10 escalating loss of control faster so that it can take over before other AIs become a threat.
In general, my impression has been that people expect multipolar worlds to often lead to cooperation between strong and weaker AIs, even if they don't align on values. I've not thought about this enough to give my opinion, but this doesn't seem like the only possible scenario.
Necessary Properties for Every AGI Safety Plan
All AGI Safety plans must have these problems (necessary properties):
- Good world model
- Good reasoning
- Specification of the values and ethics to follow
All of these require good capabilities, meaning capabilities and alignment are intertwined. Note that Shane says in the talk that you need a good world model and good reasoning to properly understand the specified values and ethics.
Shane thinks future models will solve conditions 1 and 2 at the human level. That leaves condition 3, the specification of the values and ethics to follow, which he sees as solvable if you want fairly normal human values and ethics.
Shane basically thinks that if the above necessary properties are satisfied at a competent human level, then we can construct an agent that will consistently choose the most value-aligned actions. And you can do this via a cognitive loop that scaffolds the agent to do this.
Tweet thread. Post on LessWrong where people are commenting on the properties.
Current LLMs are not worrisome, Autonomous Agents are
I keep going back to this thread on how the next AI paradigm is what we should be worried about (autonomous agents) if anything. Here's a summary:
As language models become more capable, they get better at exploiting reward models used in reinforcement learning. This requires constantly collecting more preference data and retraining reward models to avoid nonsensical outputs that have exploited the idiosyncrasies in the preference data.
More capable RL agents are better at finding loopholes in reward functions to maximize rewards in unintended ways. This is supported by intuition as well as research papers.
The current paradigm of language models themselves is not necessarily dangerous. The real risk comes from fine-tuning LLMs into autonomous agents (AutoGPTs) that have memory, can take actions, and try to maximize rewards. The widespread proliferation of GPT-4+ level models will likely lead to many such agents that could cause significant damage.
Aligning these advanced autonomous agents will be very difficult. Simply trying to trade off their reward objective with a "be nice" objective won't work, as they will find loopholes in the "be nice" objective to maximize the hard reward instead.
Unlike humans, AI systems are highly mutable. So even if we find a good balance of hyperparameters for goal-seeking vs friendliness, it would be easy for bad actors to tune the system to make it more dangerous, analogous to how nuclear technology can be repurposed into weapons.
As AI systems become more autonomous, closed-loop and difficult to monitor, unexpected harmful behaviors may emerge even if monitored tests initially look good.
In summary, the key risk is that as language models scale up in capability and are used to create autonomous agents optimizing for some reward in the real world, these agents may behave in unintended and destructive ways to maximize reward that are difficult to constrain even with careful reward engineering and monitoring. The high mutability of AI makes this even riskier.
That's it for this round-up! Consider responding to any of the threads if you'd like to discuss further.